Thought leadership
-
January 26, 2024
Data as a Value Center | Podcast (Featuring Katie Bauer)
Dive into the world of data team transformation in our latest podcast episode!
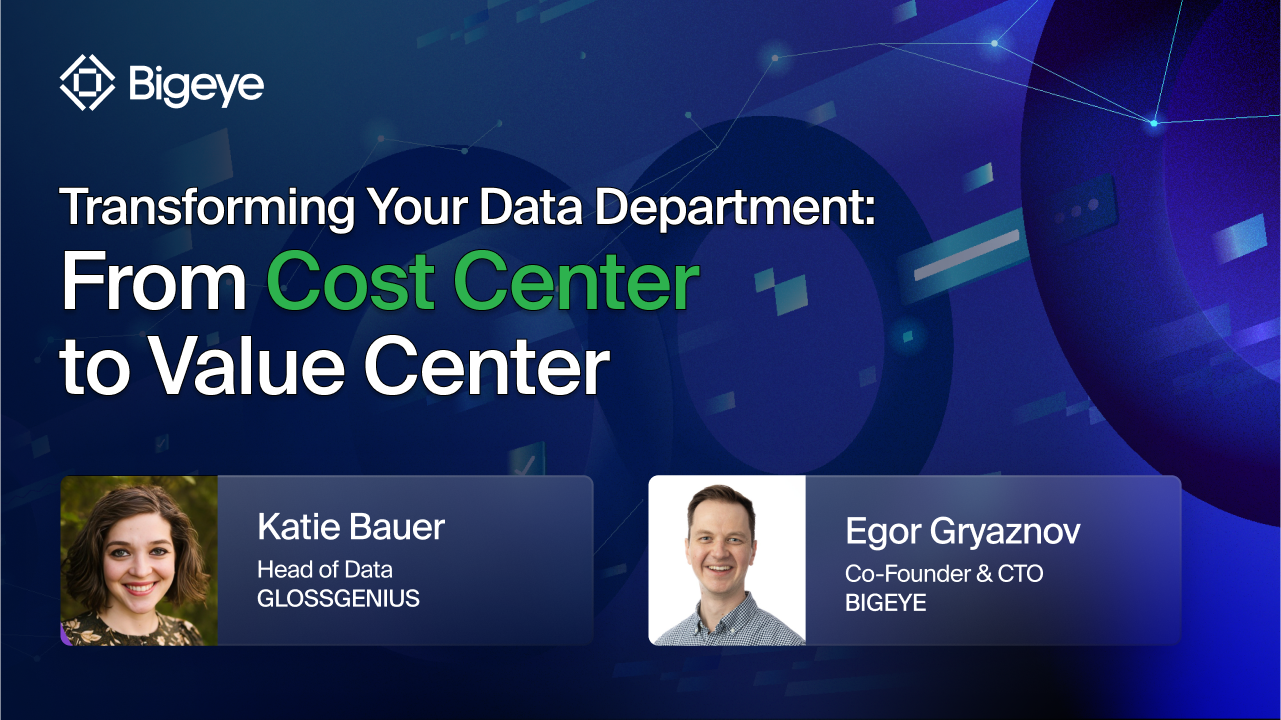
Dive into the world of data transformation in our latest podcast episode!
Discover how data departments evolve from cost centers to value centers within organizations, driving business success.
This episode dives into:
- Cost Center vs. Value Center: Explore the distinction between cost centers, focused on minimizing expenses, and value centers, striving to boost revenue and create business value.
- Team Models: Delve into two common models for structuring data teams: central data teams catering to the entire organization and hub-and-spoke models with embedded analysts and data scientists within business units.
- Challenges in Transition: Learn about the challenges data teams face during this transition, striking a balance between technical perfectionism and delivering actionable insights.
- Setting Measurable Goals: Uncover the importance of setting specific, measurable goals that align with business impact, showcasing data teams' contributions.
- ROI of Data Quality: Get insights into quantifying the ROI of data quality improvements, especially in the context of machine learning models, by measuring their impact on business metrics.
- Communication and Feedback Loops: Gain insights into bridging the gap between data teams and the organization, emphasizing clear communication, feedback loops, and aligning goals.
- Visibility and Advocacy: Learn how data teams can actively promote their work and its value, ensuring recognition within the organization.
Ready to unlock the full potential of your data strategies?
Tune in to the episode and join the transformation journey!
Listen to the podcast episode here! 🎧
share this episode
Resource
Monthly cost ($)
Number of resources
Time (months)
Total cost ($)
Software/Data engineer
$15,000
3
12
$540,000
Data analyst
$12,000
2
6
$144,000
Business analyst
$10,000
1
3
$30,000
Data/product manager
$20,000
2
6
$240,000
Total cost
$954,000
Role
Goals
Common needs
Data engineers
Overall data flow. Data is fresh and operating at full volume. Jobs are always running, so data outages don't impact downstream systems.
Freshness + volume
Monitoring
Schema change detection
Lineage monitoring
Monitoring
Schema change detection
Lineage monitoring
Data scientists
Specific datasets in great detail. Looking for outliers, duplication, and other—sometimes subtle—issues that could affect their analysis or machine learning models.
Freshness monitoringCompleteness monitoringDuplicate detectionOutlier detectionDistribution shift detectionDimensional slicing and dicing
Analytics engineers
Rapidly testing the changes they’re making within the data model. Move fast and not break things—without spending hours writing tons of pipeline tests.
Lineage monitoringETL blue/green testing
Business intelligence analysts
The business impact of data. Understand where they should spend their time digging in, and when they have a red herring caused by a data pipeline problem.
Integration with analytics toolsAnomaly detectionCustom business metricsDimensional slicing and dicing
Other stakeholders
Data reliability. Customers and stakeholders don’t want data issues to bog them down, delay deadlines, or provide inaccurate information.
Integration with analytics toolsReporting and insights